Leveraging AI for Inventory Forecasting
What is inventory forecasting and how AI is revolutionizing inventory forecasting? Explore the power of AI in transforming inventory forecasting with improved accuracy and efficiency.
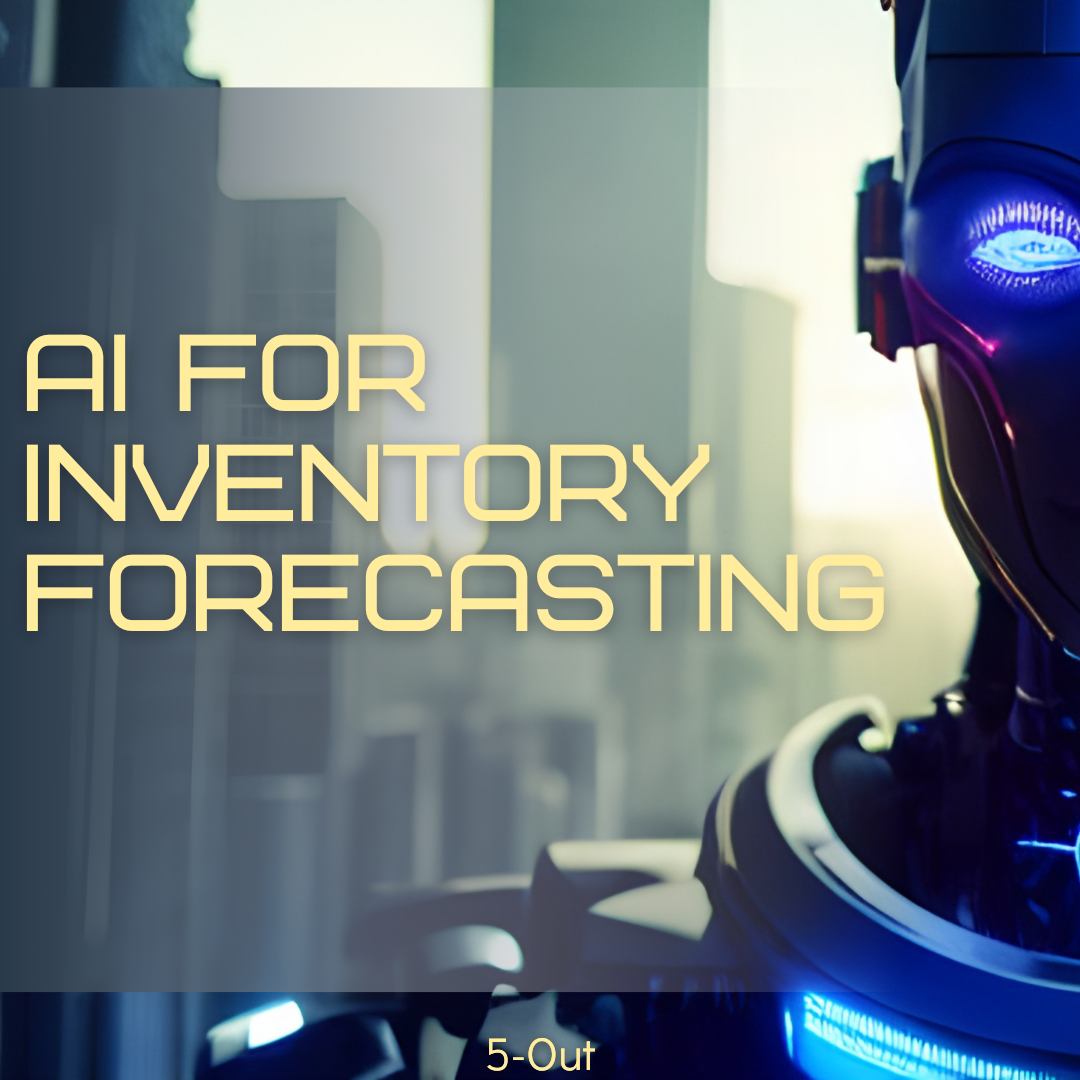
In today's digital age, the power of Artificial Intelligence (AI) has transcended traditional boundaries, reaching areas as intricate as inventory forecasting. As businesses strive to stay ahead of the curve, maintaining an optimal level of inventory planning has emerged as a decisive factor for success. The stakes are high; a surplus can lead to increased costs and waste, while a shortfall can result in missed sales and dissatisfied customers. Here's where AI steps in, transforming the landscape of inventory forecasting with remarkable precision, adaptability, and efficiency. But how exactly does AI accomplish this feat? What benefits does it offer, and what are the challenges businesses might encounter when integrating it into their systems? More importantly, how can these challenges be mitigated?

Inventory Forecasting and the Emergence of AI
Accurate inventory forecasting is a vital aspect of any business, regardless of its size. Inventory forecasting plays a crucial role in supply chains management. At its core, inventory forecasts involve predicting the number of products or materials that a business will need over a specific period. This prediction is crucial as it helps businesses maintain an optimal stock level - enough to meet inventory demand without being excessive. Excessive inventory can lead to higher storage costs and potential waste from unsold products. Conversely, inadequate stock levels can result in lost sales opportunities and unhappy customers.
In traditional inventory forecasting, methods such as time-series forecasting, causal models, replenishment average lead time in days and moving averages were typically used. While these methods served businesses adequately in the past, they often fell short in handling complex variables, sudden changes in demand patterns, and high volumes of data. This is where Artificial Intelligence (AI) comes into play, providing advanced techniques and algorithms to overcome these limitations.
AI, with its subsets like Machine Learning (ML) and Deep Learning (DL), has taken a pivotal role in modern inventory forecasting. By applying AI, companies can analyze complex, multi-dimensional data and generate forecasts considering a wide range of variables including seasonality, market trends, sales trends and consumer behavior. The predictive power of AI allows businesses to anticipate demand with a higher degree of accuracy, leading to more efficient inventory management.
The importance of AI in inventory management can't be overstated. AI-powered inventory forecasting can lead to significant cost savings by reducing stock holding and storage costs, decreasing stockouts and overstock scenarios, and improving overall operational efficiency. Additionally, AI can adapt to changing trends and make predictions in real time, a capability that is becoming increasingly essential in today's dynamic and fast-paced business environment. By leveraging AI, businesses are not just staying afloat; they are propelling themselves toward more strategic and data-driven decision-making processes.
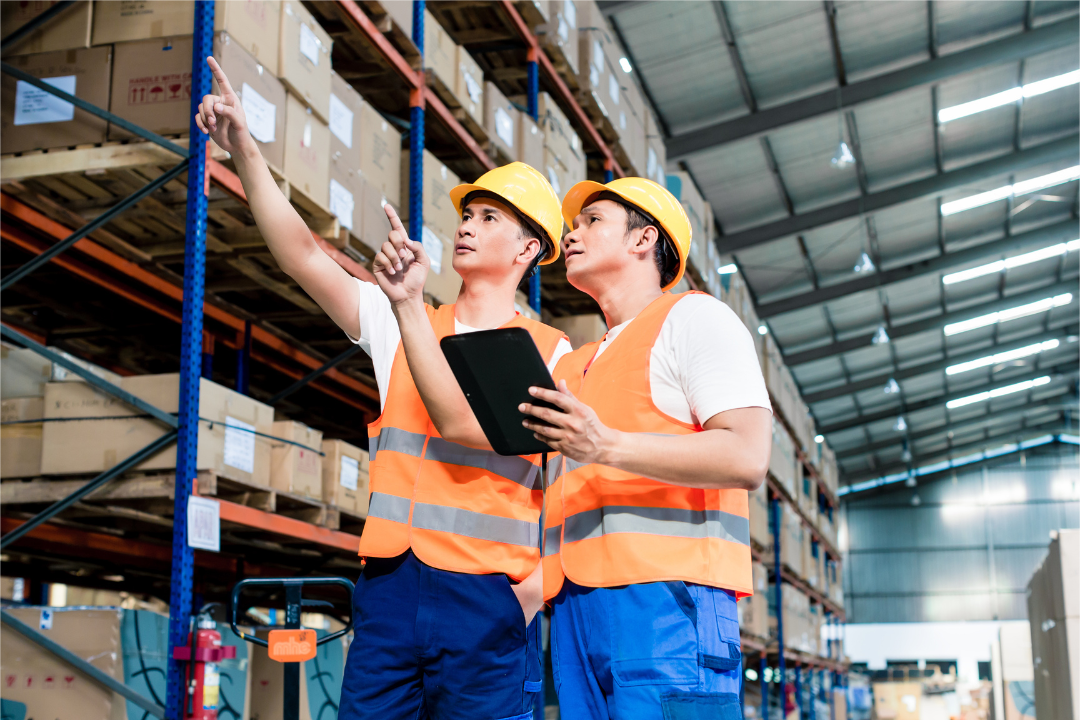
What is Inventory Forecasting
Inventory forecasting is the process by which businesses predict the amount of stock they will require over a certain period. This forecasting can range from determining the need for a specific product to predicting the requirement of raw materials for manufacturing processes. The goal is to optimize inventory levels such that a business always has just enough stock to meet customer demand, but not so much that it incurs unnecessary storage costs or risks wastage through unsold products. Both quantitative forecasting and qualitative forecasting methods are used in inventory forecasting, and their relative importance can vary depending on the specific context and availability of data.
Traditional Prediction Method
- Time-Series Forecasting: This method uses historical sales data to predict future demand. The key assumption here is that future inventory needs will follow the same pattern as the past.
- Causal Models: These methods assume that demand is influenced by certain factors or variables, such as price, seasonality, or promotional activities. Regression models are often used to establish a relationship between these factors and demand.
- Moving Averages: This method involves taking the average of sales over a specific period and using it to forecast future demand. The period can be adjusted according to the business's needs.
Limitations of Traditional Methods
While these traditional methods have been effective to some degree, they come with certain limitations.
For starters, they heavily rely on historical data and often assume that future patterns will mirror the past. This makes it challenging to adapt to sudden changes in the market or unforeseen events that significantly influence demand, like a global pandemic.
Secondly, these methods are often unable to process and analyze large volumes of data from multiple sources, something that today's businesses frequently have to deal with. Also, they may fail to consider complex interdependencies among various influencing factors, leading to inaccurate forecasts.
Lastly, these methods usually require manual intervention and expertise to set parameters, adjust models, and interpret results. This not only demands considerable time and effort but also opens the door for human error.
These limitations of traditional forecasting methods paved the way for the integration of Artificial Intelligence, which offers more advanced, accurate, and automated forecasting solutions.

The Rise of AI in Inventory Forecasting
Artificial Intelligence (AI) is a broad field that mimics human intelligence in machines. One of the crucial subsets of AI is Machine Learning (ML), where algorithms learn patterns and make predictions based on data, improving their outcomes over time without being explicitly programmed to do so. This ability to learn and adapt makes ML particularly useful for tasks such as inventory forecasting.
In the context of forecasting, AI and ML can analyze vast amounts of data from various sources, learn from this data, and predict future trends. Unlike traditional methods that rely heavily on historical data, AI can analyze complex patterns, consider a wide range of influencing factors, and adapt to changes in real time.
The Steps of AI-based Inventory Forecasting
- Data Collection: Gathering relevant data from multiple sources including past sales, real-time sales, promotional calendars, market trends, and external factors like holidays or weather conditions.
- Data Preprocessing: Cleaning and organizing the data to make it suitable for ML algorithms.
- Model Training: Feeding the processed data into ML algorithms to allow them to learn patterns and relationships.
- Prediction: Using the trained model to predict future inventory needs.
The application of AI in inventory forecasting has proven successful in various industries. For instance, Amazon uses AI extensively for inventory forecasting. Their system takes into account factors like sales history, product searches, wish lists, and even current shopping cart contents to demand planning and manage inventory efficiently.
Similarly, Walmart uses AI and ML to forecast demand for thousands of products across numerous categories, considering factors like economic indicators, weather patterns, and local events. Their AI-driven approach has resulted in more accurate forecasts, improved customer satisfaction due to fewer stockouts, and reduced wastage of unsold products.
Another example in the restaurant industry is 5-Out innovative sales forecasting software, integrated with inventory management software. Utilizing the power of AI and Machine Learning (ML), it provides restaurant owners with accurate predictions of future sales, enabling them to make informed decisions about their inventory purchasing.
The way 5-Out works is by analyzing various data points. It takes into account historical sales data but goes beyond that by integrating external variables that significantly influence the restaurant business. Factors such as local events, weather patterns, holidays, and even traffic are considered in the model. By processing these complex datasets, the software can make precise predictions about future demand.
By predicting which dishes are likely to be popular in the coming weeks, it helps restaurant owners make data-driven decisions about which ingredients to stock up on. This not only prevents waste and saves costs, but also ensures that the restaurant is always ready to serve what the customers are craving.
Book a demo today and see the power of AI and Machine Learning in action. You will know how much inventory you need and say goodbye to overstocking, food wastage, and running out of popular dishes.
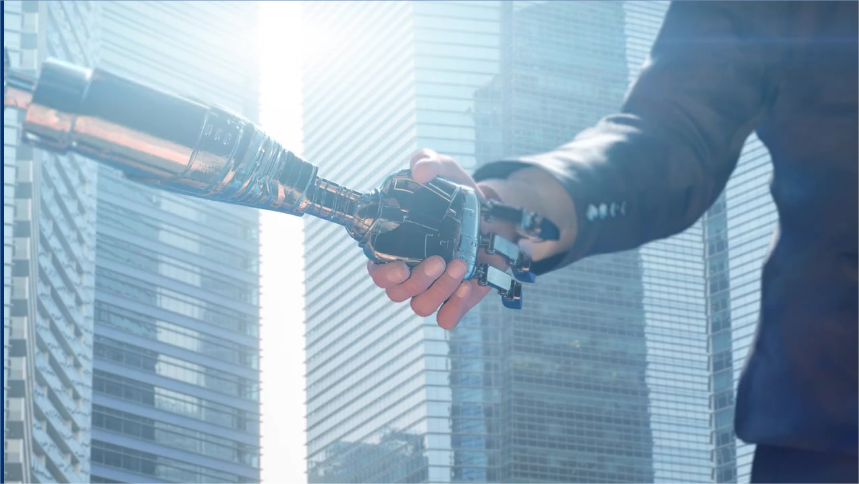
Benefits of AI in Inventory Forecasting
1. Enhanced Accuracy
AI algorithms can handle large volumes of data and complex relationships between different variables, leading to highly accurate forecasts. They consider a wide range of factors, including past sales data, seasonality, market trends, and promotional activities, to deliver predictions with a higher degree of precision than traditional forecasting methods.
2. Real-time Forecasting
AI algorithms can process data in real-time and adjust their predictions accordingly. This means businesses can respond quickly to changes in demand, helping them stay ahead of the curve and ensure they always have the right amount of stock on hand.
3. Scalability
AI-based systems can easily scale to handle an increasing amount of data as a business grows. They can also manage multiple products across different locations simultaneously, making them ideal for large businesses with extensive inventories.
4. Adaptability to Market Trends and Consumer Behaviors
AI systems can adapt to changing market trends and consumer behaviors, a capability that is becoming increasingly essential in today's dynamic business environment. By continuously learning from new data, they can update their predictions to reflect the latest trends, ensuring businesses are always prepared for what's ahead.
5. Improved Efficiency and Cost Savings
AI-driven inventory forecasting can lead to significant cost savings. By optimizing inventory levels for a future, businesses can reduce storage costs, minimize waste from unsold products, and prevent lost sales from stockouts. Furthermore, AI systems automate the forecasting process, freeing up staff to focus on other important tasks and improving overall operational efficiency.
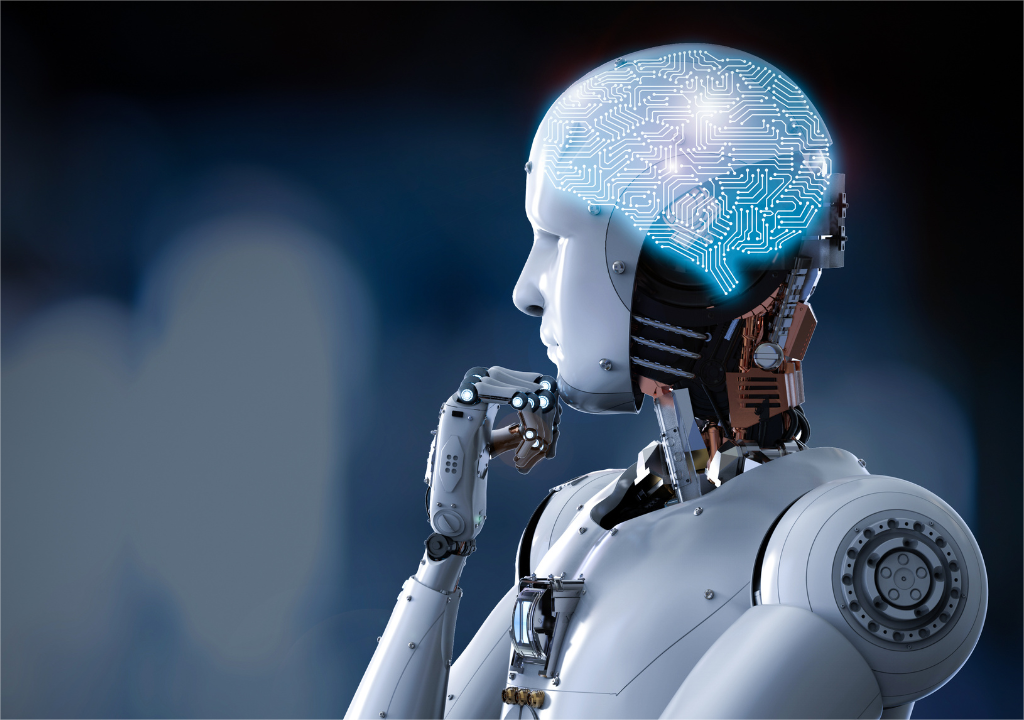
Challenges in Implementing AI for Inventory Forecasting
Despite its numerous advantages, implementing AI for inventory forecasting isn't without challenges. Here are some of the common hurdles businesses might encounter:
1. Data Quality and Management
For AI to effectively forecast inventory, it needs high-quality, accurate data. However, ensuring this can be a challenge. Data could be missing, incorrect, or outdated, and cleaning it up can be time-consuming. Furthermore, consolidating data from multiple sources into a usable format for AI algorithms can also be complex.
2. Integrating AI into Existing Systems
Integrating AI technologies with a business's existing inventory management system can be challenging. It requires careful planning, resources, and often, significant changes to the current processes. Companies also need to ensure that the AI system can communicate and interact seamlessly with the existing IT infrastructure.
3. Skill Gaps and the Need for Training
AI and ML are advanced technologies that require specific skills to implement and manage. Businesses may find it challenging to find employees with the necessary expertise. Furthermore, existing employees may need training to understand how to use and benefit from the AI system.
4. Cost and Time Investment
Implementing AI for inventory forecasting requires significant upfront investment in terms of time and money. This includes costs related to data preparation, system integration, purchasing or developing AI software, and training staff. It also takes time to train the AI model and tune it for optimal performance.
5. Trust and Understanding of AI Technology
Finally, there's often a level of apprehension or lack of understanding when it comes to AI. Some people may be skeptical of its predictions or hesitant to rely on an algorithm for crucial business decisions. Overcoming this barrier requires education and transparency about how the AI system works and the benefits it offers.
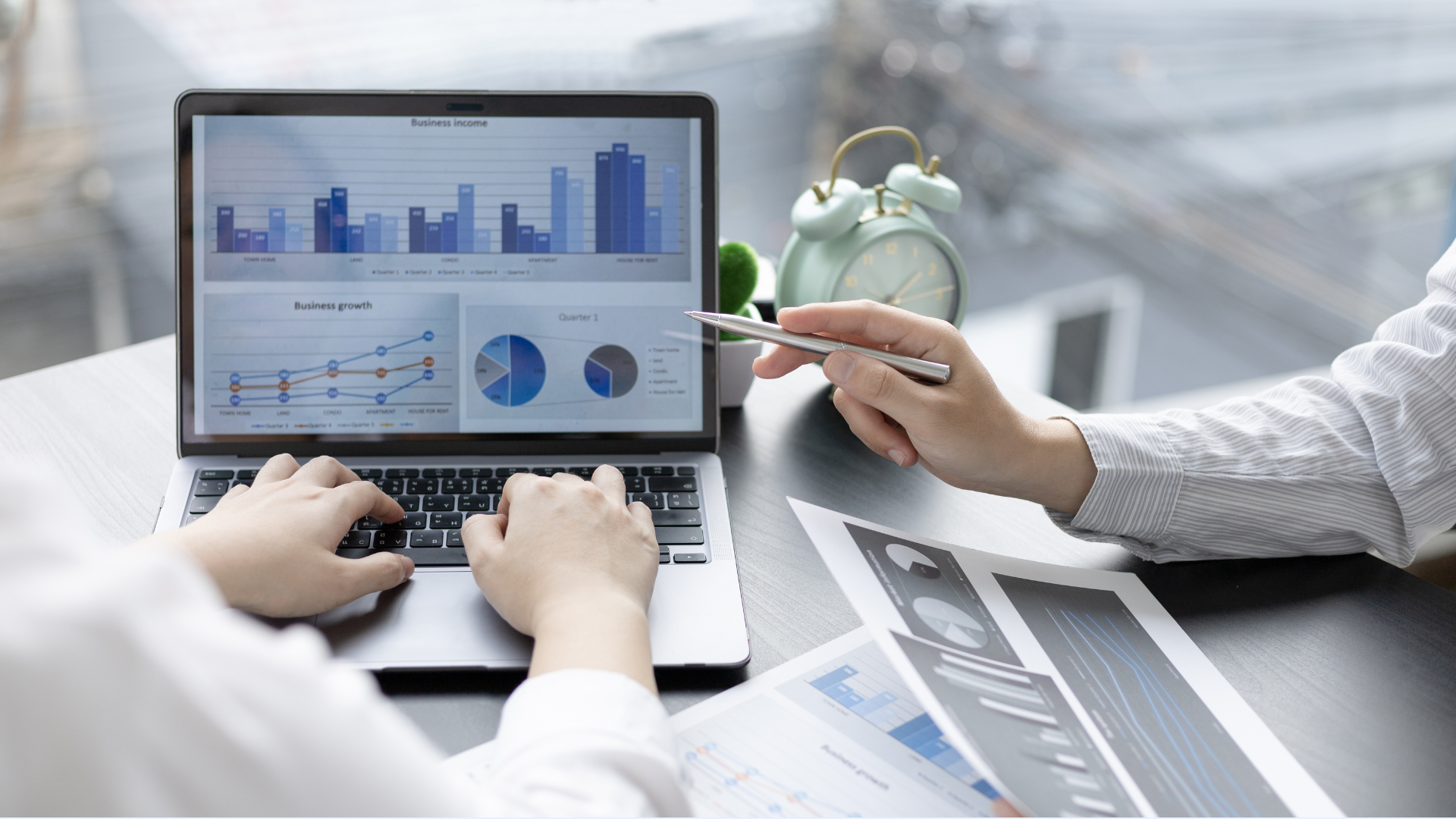
Overcoming Challenges and Implementing AI
While there are challenges in implementing AI for inventory forecasting technique, they are not insurmountable. Here are some strategies to overcome these challenges and effectively integrate AI into your inventory forecasting process:
1. Proper Data Management and Preprocessing
Data is the lifeblood of any AI system. Ensuring high-quality, relevant, and accurate data is critical. Implementing robust data management practices and preprocessing steps can help to clean, organize, and format data, making it suitable for use in AI algorithms.
2. Choosing the Right AI Tool for Your Business
Not all AI tools are created equal. Depending on the size of your business, the complexity of your inventory, and the skills of your team, some tools may be more suitable than others. Spend time researching different options, consider consulting with AI experts, and choose a tool that fits your specific needs.
3. Training and Upskilling Employees
Investing in training for your team can help bridge any skill gaps and ensure that your employees are able to effectively use the AI system. This could involve workshops, online courses, or even hiring an AI expert to provide in-house training. Remember that your team doesn't need to become AI experts, but they should understand the basics of how the system works and how to interpret its predictions.
4. Piloting the AI System Before Full Implementation
Before fully implementing the AI system, consider running a pilot project. Choose a small part of your inventory and use the AI system to forecast its demand. This can help you identify any potential issues, fine-tune the system, and gain confidence in its predictions before rolling it out on a larger scale.
5. Establishing Trust in AI
Building trust in AI takes time and transparency. Be open with your team about why you're implementing AI, how it works, and the benefits it can bring. Encourage questions and provide clear, honest answers. Over time, as people see the accuracy of the AI's predictions and the benefits it delivers, trust will grow.
By taking these steps, you can successfully overcome the challenges associated with implementing AI for inventory forecasting and unlock its many benefits. Remember that the goal is not to replace human judgment but to enhance it with powerful, data-driven insights.

In today's complex business landscape, AI has emerged as a transformative force in inventory forecasting. By analyzing vast amounts of data and adapting to real-time changes, it significantly enhances forecast accuracy and offers numerous benefits such as cost savings, improved efficiency, and scalability.
Despite the challenges in data management, system integration, skill gaps, and investment, with the right strategies, these can be effectively overcome. A notable example of this transformation is 5-Out, a restaurant sales forecasting software, which exemplifies the power of AI in driving efficient inventory management.
The time to harness the power of AI is now! Request a 5-Out sales forecasting demo today!